Problems of Lack of Transparency Pervade Issues of Algorithms in Artificial Intelligence
WASHINGTON, November 12, 2019 – The advent of artificial intelligence raises the concern of whether online algorithms harm or help user bias, experts said at a Tuesday Brookings panel. The remarkable lack of transparency is evident in how companies analyze algorithms, said Solon Barocas, information
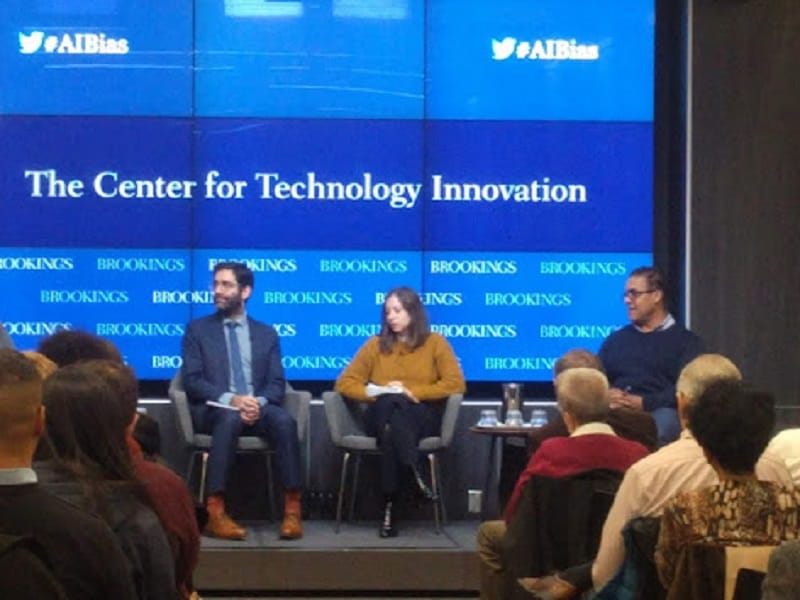
WASHINGTON, November 12, 2019 – The advent of artificial intelligence raises the concern of whether online algorithms harm or help user bias, experts said at a Tuesday Brookings panel.
The remarkable lack of transparency is evident in how companies analyze algorithms, said Solon Barocas, information science professor at Cornell University. Before technology became ubiquitous, it was easier for people to recognized blatant discrimination from companies. Now, he said, it’s more difficult to detect these signs from an online platform.
The reasons creditors provide to customers for adverse decisions, Barocas said, are not entirely useful.
The main difficulty is identifying and challenging algorithmic biases in the first place, said Center for Democracy and Technology Analyst Natasha Duarte. What experts do know, she said, is that certain user characteristics impact decisions made by online platforms.
Companies don’t know if algorithms can filter out discrimination, Duarte continued, which is why some organizations have imposed moratoriums on online recognition tools, until proper safeguards have been placed.
It’s not enough for an algorithm to have adequate accuracy, she said. These tools need to be frequently tested to ensure that they can administer appropriate results and prevent the acceptance of low-quality content.
That is especially true for facial recognition technology, as grainy image matching can have an adverse impact. There are multiple aspects to facial recognition that aren’t commonly discussed, said Karl Ricanek, computer science professor at the University of North Carolina, Wilmington.
The umbrella term of facial recognition, he said, is the process of matching a face with another in a different image. Facial analysis, on the other hand, investigate certain attributes of a person’s face to provide a more detailed description. Affective computing can also be used to analyze visible facial expressions.
Despite the vast science behind facial recognition technology, Ricanek said, members in both the public and private sectors don’t completely understand the decisions algorithms make for individuals daily.
Regulators are behind the curve, he said, because they are still trying to understand the most basic technology. Companies are also not performing the necessary due diligence to make sure their algorithms lack bias.
The bottom line, however, said Ricanek, is that current algorithms can’t tell everything that users want to know. Experts should still use common sense and analyze existing literature on the subject. Moreover, there needs to be assurance that machine learning isn’t being used to replace simple solutions that may have more political ramifications.