Siemens Highlights AI Sustainability Challenges for Data Centers
The report urges data centers to secure sustainable power sources.
Michael D. Melero
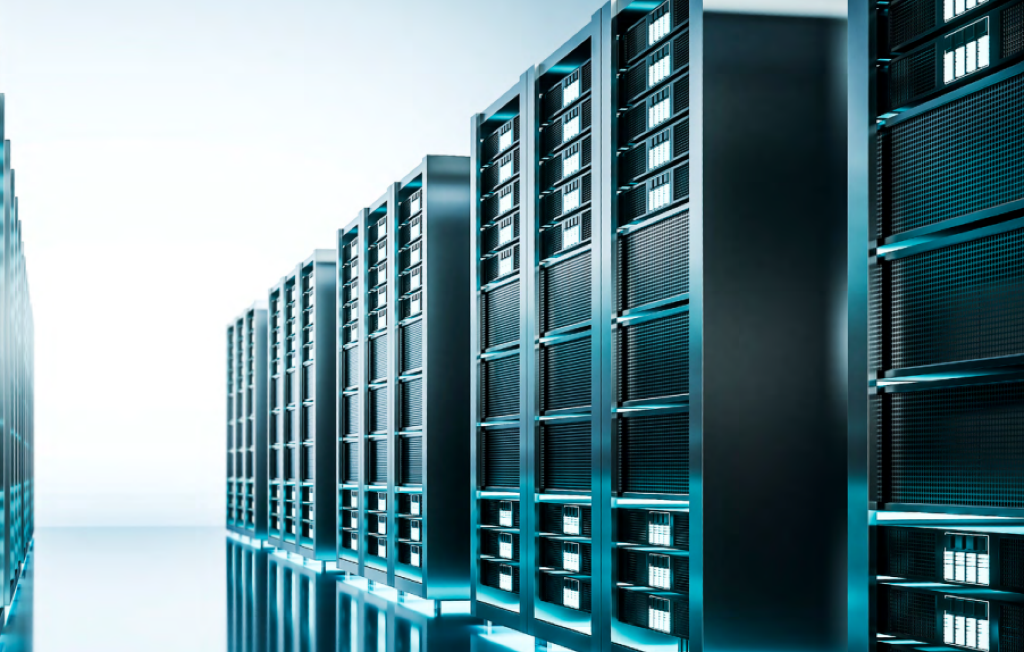
June 18, 2024 – Global technology company Siemens issued a report on Monday revealing that data centers are pushing the limits of their processing capabilities and power consumption, urging companies to secure sustainable power sources and reallocate cooling infrastructure.
Data centers currently consume between 1 to 1.5 percent of global electricity, according to the International Energy Agency. This high consumption strains their processing capabilities and power usage. Siemens suggests that to address this challenge and maintain efficiency, the data center industry must reallocate existing cooling infrastructure and secure sustainable power sources to meet high demand while upholding environmental responsibilities.
“Many major world governments have made big commitments on decarbonization, and they’ve all identified the data center industry as a key player in driving decarbonization,” said Ciaran Flanagan, global head of sales, Data Center Verticals, Siemens. “We will see more and more regulation and more need to report on overall energy consumption, energy mix, and carbon versus non-carbon. More regulation is coming,...which will drive the need for more control, more information, and more data.”
One of Siemens' solutions is the implementation of AI-driven White Space Cooling for data centers, which dynamically adjusts cooling to match IT load, optimizing energy efficiency and reducing operational costs. Additionally, connecting data centers to district heating networks allows the reuse of their waste heat, potentially reducing CO2 emissions annually.
The report identifies four key elements driven by AI and transformative technologies that are in high demand among tech companies: off-premises data centers, the Internet of Things, cloud computing, and the exponential growth of artificial intelligence.
The increasing use of AI, including Deep Learning and Large Language Models, necessitates complex model tuning and training. These technologies are primarily used for cutting-edge pattern recognition, predictive analytics, and content generation.